Will AI run out of training data by 2026?
Explore the possibility of AI exhausting its training data by 2026. Understand the implications and challenges of maintaining data availability for AI development.
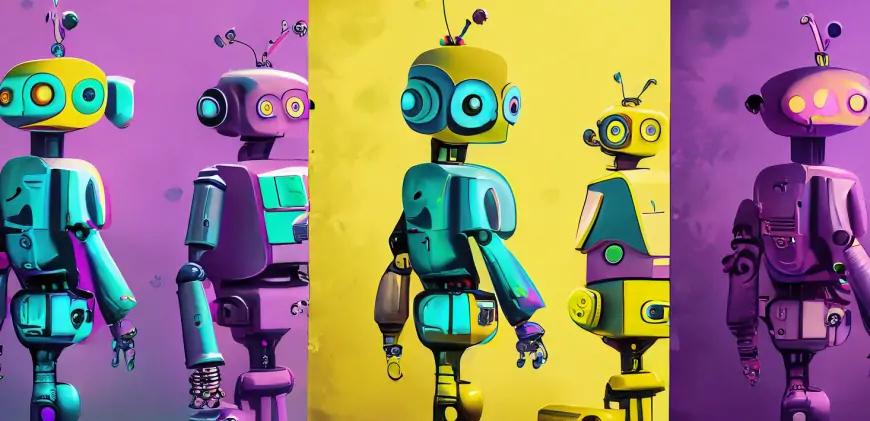
Artificial intelligence relies on data for training, operation, and evolution—it is akin to energy for AI.
The greater the quantity and quality of data, the better AI performs and improves over time.
But what if the world runs out of data?
A recently updated paper suggests that if AI development continues at its current pace, all online data could be depleted between 2026 and 2032—or even sooner if models are excessively trained.
Pablo Villalobos, the first author of the study from Epoch AI, discussed the findings with Live Science.
"If chatbots consume all available data without advancements in data efficiency, I anticipate a relative stagnation in the field. Models will only improve slowly as new algorithmic insights are found and new data is naturally generated." Should data become scarce, researchers suggest that private and synthetic data will become key solutions. However, not everyone believes this scenario will ever materialize.
"I believe that there is already sufficient data available, and future AI development will focus on enhancing learning algorithms rather than acquiring more data," Dunaev stated. He noted that the study predicts a data shortage within a few years, but the rapid pace of AI development makes long-term forecasts challenging. Additionally, he highlighted that humanity will continue to generate more data and research, with AI's assistance.
Jim Kaskade, CEO at Conversica, a Conversational AI provider, also commented on the study to Techopedia. Kaskade acknowledged the robustness and sound methodology of the study's projections but emphasized the dynamic nature of internet and data generation. He pointed out that over 2.5 quintillion bytes of data are created daily, with social platforms generating 100 trillion texts annually, 1.5 trillion tweets per year, over 260 million hours of YouTube videos uploaded annually, and over 1 trillion photos shared each year.
Dmytro Shevchenko, a Classic Machine Learning, Computer Vision, and Natural Language Processing expert, and Data Scientist at Aimprosoft, concurred with the study but pointed out that its conclusions are incomplete as they don't consider new advancements. He cited improvements in data compression algorithms and optimization techniques that may significantly reduce the need for vast amounts of data. Shevchenko also mentioned the potential of synthetic data and transfer learning, noting that the study does not fully account for the complexities and limitations of these methods.
Thousands of new AI companies emerge
The AI ecosystem is rapidly expanding, with a surge in companies developing, integrating, and applying AI technologies. This exponential growth in new AI companies is identified by the study as a factor affecting data availability and usage.
According to the global startup data platform Tracxn, there are 75,741 companies in the AI sector as of June 27. This includes both leading firms and AI startups poised for significant growth in 2024. The number of companies in this field is increasing by approximately 10% each month.
Can AI technology advance without data?
One of the study’s conclusions is that without data, AI technology advancements are not possible. Kaskade from Conversica told Techopedia that the lack of new data would hinder AI progress.
"The study highlights that LLMs rely heavily on large-scale, high-quality data for training," Kaskade explained. "Without new data, these models would struggle to learn from evolving trends and contexts, reducing their effectiveness and accuracy."
However, the study also suggests potential solutions such as synthetic data generation, transfer learning from data-rich domains, and improvements in data efficiency.
While Kaskade expressed reservations about synthetic data, he acknowledged that it could help maintain AI development momentum by providing alternative data sources, even in the absence of new human-generated data.
"If AI were to run out of data due to resource constraints or otherwise, I would assume providers would simply purge the old data to capture the new — aside from models trained specifically on prior periods, which require no recent data to perform their tasks."
If synthetic data, learning transfers, and the private data industry fail to meet the demands of future AIs, the technology will reach a performance plateau, Kaskade warned. This would be similar to model drift, where a model’s performance degrades over time as the data it was trained on becomes outdated or irrelevant.
"This would result in models becoming less effective over time as they fail to incorporate new information and trends. Additionally, the absence of fresh data could lead to overfitting, where models become too specialized on existing data and perform poorly on any new tasks."
Dunaev from Comply Control believes the answer lies in optimizing algorithms rather than acquiring more data. "Given the current pace of development and AI’s capability to generate new data and research, a lack of data is not a significant limitation for future progress," Dunaev said.
"If AI does run out of data, it will still improve by optimizing learning algorithms and conducting its own research to gather new data. So, even with limited data, AI will be able to continue growing and improving."
Shevchenko from Aimprosoft is uncertain whether AI models will evolve smoothly in a data crisis.
"Real data is the backbone of AI development, providing diverse, rich, and contextually relevant information that allows models to learn, adapt, and generalize efficiently to different scenarios," Shevchenko said.
"Synthetic data generation, transfer learning, and data optimization techniques can mitigate the impact of data scarcity. However, these methods cannot fully replace the richness and contextual relevance of real data."