What are AI chips? Revolutionizing computing power for Artificial Intelligence
Explore the future of AI chips, including challenges like supply chain issues, geopolitical tensions, and the demand for more advanced computational power.
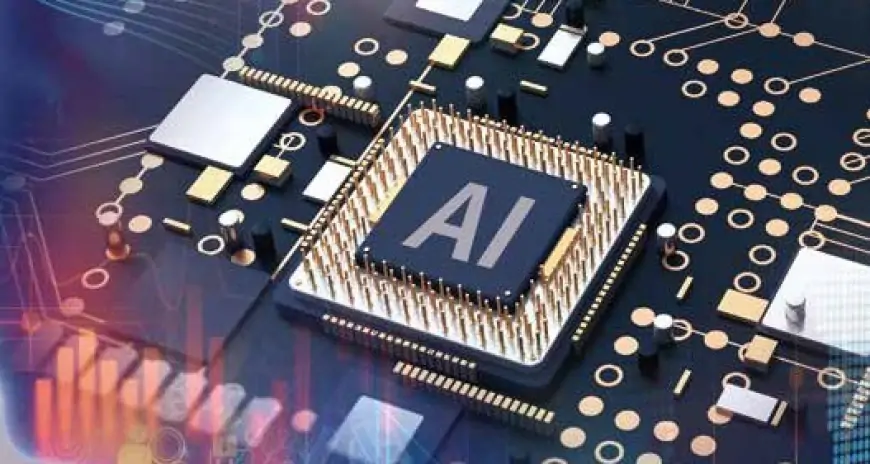
AI chips refer to specialized computing hardware designed to support the development and deployment of artificial intelligence systems. As AI continues to evolve, the demand for higher processing power, speed, and efficiency in computing has also increased — and AI chips play a crucial role in meeting these requirements. Many AI breakthroughs in recent years — from IBM Watson’s historic Jeopardy! win to Lensa’s viral social media avatars and OpenAI’s ChatGPT — have been driven by AI chips. To continue advancing technologies like generative AI, autonomous vehicles, and robotics, the evolution of AI chips will remain essential.
“As the cutting edge keeps moving and keeps changing,” said Naresh Shanbhag, an electrical and computer engineering professor at the University of Illinois Urbana-Champaign, “then the hardware has to change and follow, too.”
Understanding AI chip
The term “AI chip” broadly refers to various chips designed to efficiently handle the complex computational demands of AI algorithms. This includes graphics processing units (GPUs), field-programmable gate arrays (FPGAs), and application-specific integrated circuits (ASICs). While central processing units (CPUs) can manage simpler AI tasks, they are becoming less effective as the field progresses.
Understanding how AI chips function
A chip, generally speaking, is a microchip or an integrated circuit made from semiconductor material at a microscopic scale. This material contains components like transistors—tiny switches that control electrical current within circuits. While memory chips manage data storage and retrieval, logic chips handle the processing of that data.
AI chips primarily focus on logic processing, managing the heavy data processing demands of AI workloads, which go beyond the capabilities of general-purpose chips like CPUs. They are built with a large number of smaller, faster, and more efficient transistors, enabling them to perform more computations per unit of energy. This design results in faster processing speeds and lower energy consumption compared to chips with larger, less efficient transistors.
AI chips also include special features that significantly speed up the computations required for AI algorithms, such as parallel processing. Parallel processing enables AI chips to perform multiple calculations simultaneously, which is essential for handling the complex computations AI workloads require. As Hanna Dohmen, a research analyst at Georgetown University’s Center for Security and Emerging Technology (CSET), explains, “AI chips are particularly effective for AI workloads and training AI models.”
GPUs, FPGAs, ASICs, and NPUs
Different types of AI chips are tailored to varying hardware designs and functionalities:
- GPUs: Originally created for high-performance graphics tasks like gaming and video rendering, GPUs are now commonly used for training AI models. Due to the computational intensity of AI training, multiple GPUs are often connected to work together, allowing for faster training times.
- FPGAs: These chips excel in applying AI models because they can be reprogrammed "on the fly," making them highly specialized for different tasks like image and video processing. Their flexibility allows them to be highly efficient in a variety of AI applications.
- ASICs: These are custom-built accelerator chips designed for a specific use, such as artificial intelligence. Since ASICs are optimized for a particular task, they offer superior performance compared to general-purpose processors or even other AI chips. Google’s Tensor Processing Unit (TPU) is a well-known example of an ASIC designed to enhance machine learning performance.
- NPUs: These specialized chips enable CPUs to manage AI workloads and are designed for deep learning and neural networks. NPUs excel at processing large amounts of data for tasks like object detection, speech recognition, and video editing, often outperforming GPUs in specific AI processes.
Why AI chips outperform traditional chips
AI chips offer significant advantages over general-purpose chips in AI development and deployment due to their specialized design features.
- Parallel processing capabilities: Unlike general-purpose chips like CPUs that rely on sequential processing (handling one task at a time), AI chips utilize parallel processing, enabling them to perform multiple calculations simultaneously. This allows large, complex problems to be broken down and solved more efficiently, resulting in faster processing speeds.
- Greater energy efficiency: AI chips are engineered to consume less energy than standard chips. Some use techniques like low-precision arithmetic, which allows computations to be completed using fewer transistors and therefore less energy. By distributing workloads efficiently through parallel processing, AI chips help minimize energy consumption. This energy efficiency is particularly important in reducing the carbon footprint of data centers and optimizing battery life in edge AI devices, such as smartphones.
- Customizability: AI chips such as FPGAs and ASICs can be customized to suit specific AI models or applications, enabling the hardware to adapt to various tasks. Developers can fine-tune parameters or optimize the chip's architecture for specific AI workloads, making it easier to accommodate different algorithms, data types, and computational needs. This flexibility is key to advancing AI technologies.
- Enhanced accuracy: Designed specifically for AI tasks, AI chips excel in performing complex computations involved in AI algorithms with greater accuracy. This leads to better performance in areas like image recognition and natural language processing, making them ideal for critical applications like medical imaging and autonomous vehicles, where precision is vital.
AI chip applications
Modern artificial intelligence wouldn’t be feasible without the use of specialized AI chips. Below are some key ways these chips are utilized.
- Large Language Models (LLMs): AI chips accelerate the training and optimization of machine learning and deep learning algorithms, which is crucial for the development of LLMs. By enabling parallel processing for sequential data and optimizing neural network functions, AI chips significantly enhance the performance of LLMs, supporting generative AI tools like chatbots, AI assistants, and text generators.
- Edge AI: AI chips enable AI processing directly on smart devices — including watches, cameras, and household appliances — in a process called edge AI. This allows data processing to occur closer to its source, reducing latency while improving security and energy efficiency. These chips are vital for applications ranging from smart homes to smart cities.
- Robotics: AI chips are integral to machine learning and computer vision tasks in robotics, enhancing robots' ability to perceive and interact with their surroundings. This is beneficial across various types of robots, from cobots harvesting crops to humanoid robots offering companionship.
- Autonomous vehicles: AI chips play a crucial role in advancing the intelligence and safety of driverless cars. They process vast amounts of data from the vehicle’s sensors, like cameras and LiDAR, facilitating tasks such as image recognition. With their parallel processing capabilities, AI chips enable real-time decision-making, allowing vehicles to navigate complex environments, detect obstacles, and adapt to changing traffic conditions.
The outlook for AI chips
While AI chips are pivotal in advancing artificial intelligence, their future faces several challenges, including supply chain issues, geopolitical tensions, and computational limitations.
- Monopoly concerns: Nvidia currently dominates the AI hardware market, controlling about 80% of the global GPU share. However, this dominance has raised antitrust concerns, as Nvidia, alongside Microsoft and OpenAI, has faced scrutiny for potentially violating U.S. antitrust laws. Recently, the startup Xockets accused Nvidia of patent theft and antitrust violations due to its acquisition of Mellanox, which could significantly impact the AI chip industry if Nvidia is found guilty.
- Supply chain bottlenecks: Taiwan Semiconductor Manufacturing Corporation (TSMC) produces about 90% of the world’s advanced chips, including Nvidia’s H100 and A100 processors. TSMC’s dominance has caused supply chain bottlenecks, as demand for AI chips exceeds supply. However, TSMC is working to expand production with new factories in Japan and the U.S. Meanwhile, tech giants like Microsoft, Google, and Amazon are developing their own AI chips to reduce dependence on Nvidia. Additionally, companies like Intel and Qualcomm are introducing alternatives, aiming to challenge Nvidia’s market position.
- Computational constraints: As AI models become larger and more complex, the computational demands on AI chips continue to rise. However, AI chips have finite computational power, and the number of chips required to support state-of-the-art AI systems is growing rapidly. To address this, companies are exploring innovations in AI hardware, such as in-memory computing, which combines data storage and processing to enhance speed. Chipmakers like Nvidia and AMD are also integrating AI algorithms to optimize performance and keep pace with AI advancements.
- Geopolitical risks: Taiwan's importance in the AI chip industry makes it a focal point in geopolitical tensions, particularly with China, which considers Taiwan a rogue province. A potential Chinese invasion could disrupt TSMC’s operations and threaten global AI chip supply. Further complicating matters, the U.S. has imposed export controls limiting China’s access to advanced AI chips, contributing to the ongoing U.S.-China tech rivalry.