How do AI chatbots work: Explained by Digimagg
Discover how AI chatbots function, from understanding natural language to generating responses. Explore their capabilities and limitations.
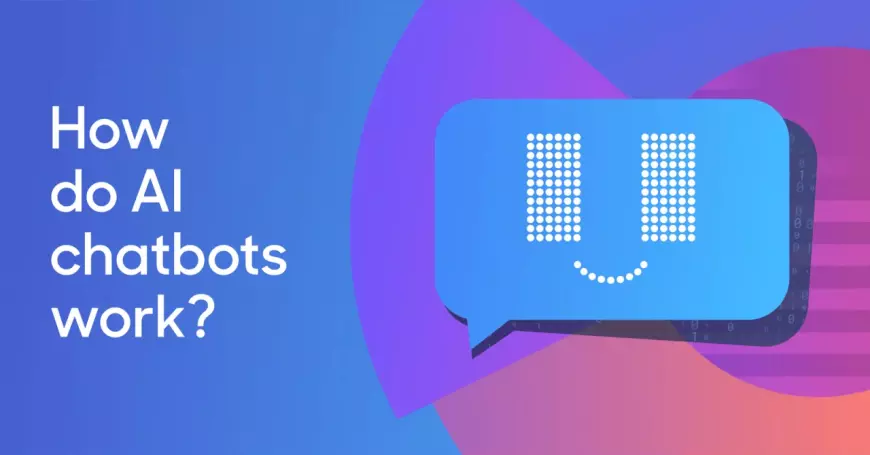
AI chatbots are currently a popular subject, but have you ever thought about how they operate? We'll delve into the technology behind these AI bots, discussing their significant potential as well as their constraints. This will provide you with a more profound comprehension of these valuable digital tools.
Chatbots have evolved significantly since their early experiments in the 1960s, including notable trials like Microsoft's Clippy assistant, which debuted with Office 97 and was retired with Office XP in 2001. With advancements in AI and Natural Language Processing (NLP), chatbots have become increasingly sophisticated. They can now understand and respond genuinely to human language. But, let's start with the basics: what exactly is an AI chatbot?
Definition of an AI chatbot
In straightforward terms, a chatbot is a software program that interacts with humans using Artificial Intelligence (AI) technologies like Natural Language Understanding (NLU) and Machine Learning. Picture an AI chatbot as a virtual assistant with whom you can converse in a two-way dialogue. It comprehends human language, interprets your queries, and provides meaningful responses. Now, let's explore how they operate behind the scenes.
How AI Chatbots Function
AI chatbots operate by combining various technologies into a cohesive, multi-layered system.
Machine Learning (ML)
Machine learning can be likened to a set of rules or instructions, known as algorithms, that guide the chatbot's learning from data, enabling it to make decisions without explicit programming. These rules are crucial for the chatbot to understand the words used in a conversation.Additionally, a chatbot has a memory mechanism, allowing it to store information from conversations. With each interaction, the chatbot's language skills grow and develop.
Similar to learning a new language, the chatbot improves its language proficiency by conversing with more people, enabling it to understand more words and phrases, and respond more accurately.When the AI chatbot encounters mistakes or misunderstandings, it learns from them and adjusts for future interactions, leading to continuous improvement in understanding human language.Machine learning algorithms come in various types, but they generally fall into two categories: supervised learning and unsupervised learning.
Supervised learning: involves training an algorithm using a dataset that includes input-output pairs. For instance, an algorithm might be trained on labeled images to recognize objects in new images.
Unsupervised learning involves using a dataset without labels or output variables to identify patterns or structures, such as grouping similar examples. For instance, an algorithm might group customers into segments based on their buying history.Despite the sophistication of machine learning technology, ML algorithms are not always 100% accurate and have limitations.
Natural Language Processing (NLP)
Fundamentally, Natural Language Processing (NLP) is a technology that aids computers in comprehending and processing human language. It is utilized by chatbots and AI programs to interpret the words and phrases used in conversations.
NLP encompasses several tasks, including:
- Parsing and part-of-speech tagging: Understanding sentence structure and the role of each word, such as identifying subjects and verbs.
- Intent Recognition: Identifying the intention behind a person's words, determining what they want or intend to do.
- Tokenisation: The process of dividing a sentence into individual words or phrases.
- Entity Extraction: Identifying and categorizing entities like dates, postal codes, and amounts from longer texts.
- Sentiment Analysis: Analyzing the sentiment expressed by a person during a conversation.
These processes collectively enable AI to comprehend the meaning of sentences and respond appropriately. With more data and advanced technology, AI can better understand and generate human language. Another fascinating area is Machine Learning.
Neural linguistics in AI
Neural Linguistics is a discipline that merges Natural Language Processing with neural networks to empower computers to comprehend and generate human language. It is crucial for AI chatbots as it enables them to engage in conversations with people in a manner similar to humans. Neural Linguistics equips AI with the capability to grasp the context, intent, and sentiment behind human speech, which is essential for generating responses that sound natural.
Generalist Large Language Models like ChatGPT are well-suited for broad conversations but have a critical flaw - they hallucinate about 15%-20% of the time, meaning they fabricate facts when they don't know the answer. This is unacceptable in industry use cases where accuracy is paramount.
This is where Custom Language Models excel. These models are trained on industry-specific data, making them highly specialized and precise. For instance, a Custom Language Model used for AI chatbots in the credit and collections industry would require training on millions of real-life customer conversations to effectively communicate in the language of credit and collections.
Large Language Models (LLMs)
Large Language Models (LLMs) are a form of AI designed to comprehend and produce natural language text. They rely on deep learning techniques, which involve training a neural network using a vast dataset.
The core concept of an LLM is to provide the AI with access to extensive text data, such as books and websites. The AI then uses this information to grasp the patterns and connections between words and phrases, a process known as "training the model".The model's accuracy and complexity improve with the amount of data it is trained on. Additionally, ongoing fine-tuning with new data can further enhance the model's performance.
Types of AI chatbots
First, let's consider basic rule-based chatbots, akin to diligent students. They strictly adhere to a set of rules to determine their responses, making them suitable for simple tasks and specific questions, but they have limited flexibility.
On the other hand, self-learning AI chatbots are like clever students who continuously improve. They utilize AI to enhance their responses over time, learning from past conversations and adapting to new scenarios. This puts them ahead of rule-based chatbots, as they can understand context, intent, and respond to more varied questions.
Chatbot architecture refers to the underlying structure and design of a chatbot, determining how it processes text. Several types exist, including:
- Rule-based chatbots, which adhere strictly to predefined logical paths.
- Retrieval-based chatbots, akin to encyclopedias, with a database of pre-written responses. They select the closest matching response, but struggle with entirely new queries.
- Generative chatbots, resembling creative writers, use neural networks to generate responses. They are trained on extensive datasets and can produce new answers, but may sometimes provide nonsensical responses if not trained properly.
- Hybrid chatbots, versatile and adaptive, combine predefined rules, responses, and neural networks to generate the most suitable response. They blend the structured approach of rule-based chatbots with the creativity of generative chatbots.
Integrating with messaging channels
AI chatbots can be incorporated into different messaging platforms to digitally engage with customers through their preferred channels, such as WhatsApp, SMS, and Messenger. Integration usually entails linking the chatbot to the messaging platform's API, enabling it to send and receive messages via these channels. This application of AI chatbots is revolutionizing customer service, particularly in contact centers.
Advantages and limitations of AI chatbots
Advantages:
- 24/7 availability
- Cost-effectiveness
- Personalization
- Ability to handle multiple queries simultaneously
- Capability to address a wide range of questions
- Automation of repetitive tasks
- Conversations that sound natural
Limitations:
- Limited ability to understand emotions
- Difficulty in handling complex requests
- Reliance on accurate data
However, AI chatbots can occasionally make mistakes. Their effectiveness is contingent on the quality of the data and algorithms used in their training; thus, if the data is flawed, the chatbot's responses may be inaccurate. Additionally, they cannot address every question or manage every scenario, indicating that there are still constraints on their capabilities.
Conclusion
AI chatbots are becoming increasingly intelligent and valuable. As technology advances, these chatbots are improving their ability to comprehend human language and provide genuinely useful responses. Currently, they are effectively used in customer service, as personal digital assistants, and in e-commerce. However, in the future, they will become even more powerful and will play a larger role in automation, allowing people to concentrate on more critical tasks. Overall, the future of chatbots appears promising.