Google AI forecasts long-term climate trends and weather in minutes
Google AI forecasts long-term climate trends and weather with high precision, offering insights into future patterns and helping plan for a sustainable future.
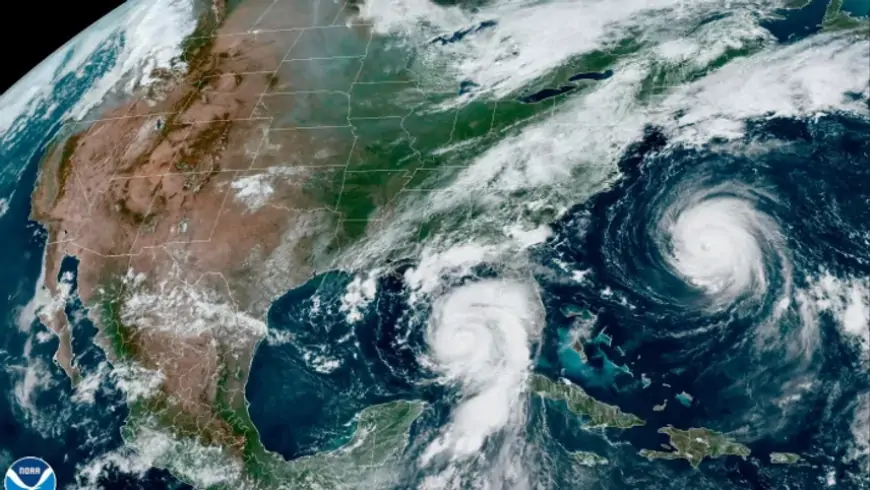
A new computer model that merges traditional weather-forecasting techniques with machine learning has surpassed other AI-based tools in predicting both short-term weather scenarios and long-term climate trends. This innovative model, featured in Nature on July 22, is the first machine-learning tool capable of producing accurate ensemble forecasts, which offer a range of possible scenarios. It promises faster and more energy-efficient forecasting compared to current methods, while providing greater detail than models relying solely on AI.
Study co-author Stephan Hoyer from Google Research highlights that unlike traditional climate models, which require supercomputers, this new model can deliver forecasts in minutes. While general circulation models (GCMs) use physics to simulate Earth's atmospheric and oceanic processes and predict weather and climate, they demand substantial computing power. Advances in machine learning, however, are making it possible to create more efficient forecasting models by leveraging extensive historical weather data.
Existing machine-learning models, such as Huawei's Pangu-Weather and DeepMind's GraphCast, offer similar accuracy to traditional GCMs for single forecasts but fall short in ensemble forecasting and long-term climate predictions. Scott Hosking from the Alan Turing Institute points out that while machine learning models are trained on existing data, they need to extrapolate into the future as climate conditions evolve. Incorporating physical principles into these models helps ensure they remain realistic and accurate.
Hybrid model
Hoyer and his team have developed NeuralGCM, a hybrid model that integrates traditional physics-based atmospheric solvers with AI components. NeuralGCM was used to generate both short- and long-term weather forecasts, as well as climate projections. The team evaluated its performance by comparing its predictions with real-world data and outputs from other models, including general circulation models (GCMs) and purely machine-learning-based tools.
NeuralGCM performed well in providing accurate short-term forecasts (one to three days ahead) while using significantly less power than GCMs. It also showed fewer errors in long-term forecasts (beyond seven days) compared to other machine-learning models. Its long-term predictions were on par with those of the European Centre for Medium-Range Weather Forecast’s ensemble model (ECMWF-ENS), which is considered a benchmark in weather forecasting. Additionally, NeuralGCM excelled at forecasting specific weather phenomena like tropical cyclones, outperforming both pure machine-learning models and ultra-high-resolution global storm-resolving models in terms of accuracy and realism.
Scott Hosking emphasizes the importance of accurate forecasting for improving decision-making and preparedness. Hoyer and his team plan to continue refining NeuralGCM by incorporating more aspects of Earth science to enhance its accuracy and effectiveness in future versions.
What's Your Reaction?
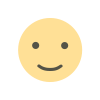
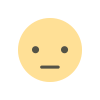
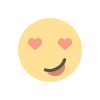
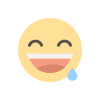
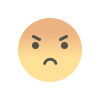
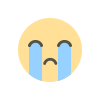
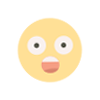