What is AI bias and how to avoid it? Definition from Digimagg
Understanding AI bias is crucial for responsible AI development. Learn what AI bias is, its impacts, and how to mitigate it in this comprehensive guide.
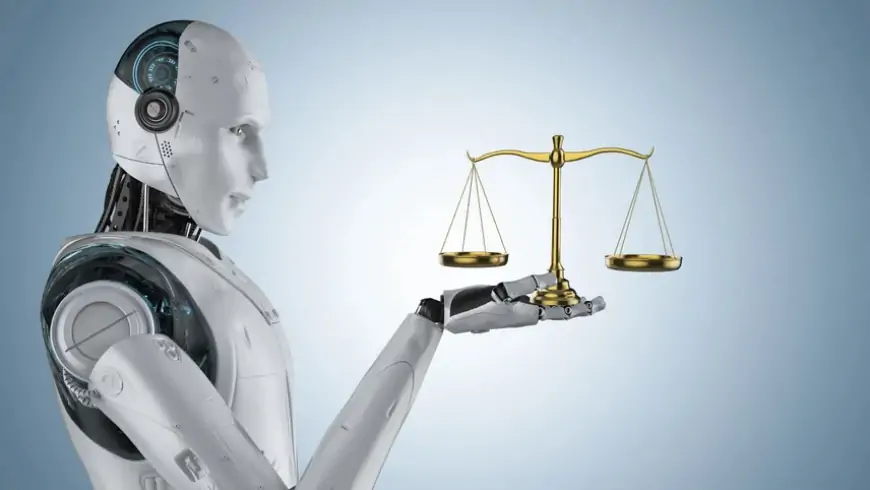
Algorithms exhibit biases when they assess people, events, or objects differently for different purposes. It's crucial to grasp these biases to develop solutions for building unbiased AI systems. This article will explore AI bias, its types, examples, and strategies to mitigate it. Let's start with defining AI bias.
What is AI bias?
Machine Learning bias, also referred to as algorithm or Artificial Intelligence bias, describes the tendency of algorithms to mirror human biases. It occurs when an algorithm consistently produces biased outcomes due to flawed assumptions in the Machine Learning process. In today's environment, characterized by a push for greater representation and diversity, this issue becomes particularly concerning as algorithms might inadvertently reinforce biases.
For instance, a facial recognition algorithm might be better at recognizing white individuals than black individuals because it has been predominantly trained on data featuring more white faces. This can have detrimental effects on minority groups, perpetuating discrimination and hindering equal opportunities. The challenge lies in the unintentional nature of these biases, making them difficult to identify until they are embedded in the software.
3 AI bias examples
As AI technology pervades various aspects of our lives, ensuring its fairness and impartiality is crucial. AI bias can lead to real-world consequences, such as unfair treatment and inaccurate decisions. While AI offers significant benefits, understanding its biases is essential before deploying AI systems.
Procedural fairness, or its absence, can significantly impact different industries:
- Financial services: AI is increasingly used in financial firms for loan approvals and credit ratings. Biased algorithms may unfairly deny loans or provide inaccurate credit ratings. For example, an AI algorithm trained on data primarily from one racial group could unfairly deny loans to individuals from other racial groups.
- Education system: AI is employed in student admissions, where biased algorithms may unfairly accept or reject students based on their backgrounds. For instance, an AI trained on biased data may favor certain genders or races in admissions decisions.
- Law enforcement: Facial recognition technology's misidentification can lead to wrongful arrests. Biased facial analysis algorithms may result in false positives, particularly affecting people of color who are already disproportionately represented in arrests.
Addressing AI bias is crucial for creating trustworthy AI systems that benefit society as a whole.
How does AI bias mirror the biases present in society?
Unfortunately, AI is not immune to human biases. While it can help humans make more impartial decisions, this is only possible if we work actively to ensure fairness in AI systems. The root cause of AI bias often lies in the underlying data rather than the method itself. Here are some key findings from a McKinsey study on addressing AI bias:
- Models can be trained on data that reflect human choices or societal disparities. For example, word embeddings trained on news articles may exhibit gender biases prevalent in society.
- Data can be biased due to how it is collected or selected for use. In criminal justice AI models, oversampling specific areas may result in more data on crime in those areas, potentially leading to biased enforcement.
- User-generated data can create a bias feedback loop. For instance, searches containing the term "arrest" were more likely to appear for African-American-identifying names compared to white-identifying names, potentially due to user interactions with search results.
- Machine Learning systems may identify statistically significant correlations that are socially inappropriate or illegal. For example, a mortgage lending model might erroneously conclude that older people are more likely to default, leading to age discrimination.
A notable real-world example is the Apple credit card issue, where male applicants were offered significantly higher credit limits than female applicants, highlighting the dangers of gender-based bias in AI algorithms.
How can we address the biases in AI?
Here are some proposed solutions:
- Testing algorithms in real-life scenarios: When using AI for tasks like selecting job applicants, it's crucial to test the algorithm with diverse candidate pools. This helps identify and address biases that may arise when applying the algorithm to groups not well-represented in the training data.
- Considering counterfactual fairness: Fairness in AI can be complex and context-dependent. Researchers have developed methods to ensure AI systems adhere to fairness criteria, such as pre-processing data, adjusting the system's decisions post hoc, or incorporating fairness definitions into the training process. Counterfactual fairness, for instance, ensures that an AI's decisions would remain the same in a hypothetical world where sensitive attributes like race or gender are changed.
- Implementation of Human-in-the-Loop systems: Human-in-the-Loop technology aims to address problems that neither humans nor computers can solve independently. This approach creates a feedback loop where humans intervene when machines encounter issues, leading to improved performance and accuracy over time.
- Reform of science and technology education: Craig S. Smith suggests a major change in how people are educated about technology and science. He argues for a reform that promotes multidisciplinary collaboration and a reevaluation of educational approaches. Issues should be addressed globally or locally, similar to the FDA's role in regulating food and drugs. Principles, standards, regulatory bodies, and public input should be part of algorithm verification processes. Simply diversifying data collection is not sufficient to solve problems; it is just one aspect of a comprehensive approach.
Will these solutions address all the issues?
While these adjustments would be advantageous, some issues may necessitate more than technological solutions and require a multidisciplinary approach, incorporating insights from ethicists, social scientists, and other humanities scholars.
Furthermore, these changes alone may not suffice in scenarios such as assessing the fairness of a system for release or determining whether fully automated decision-making should be allowed in certain contexts.
Will AI ever be free of bias?
The simple answer? Yes and no. Achieving a completely unbiased AI is theoretically possible, but practically challenging. Just as it's unlikely for a perfectly unbiased human mind to exist, an AI's impartiality depends heavily on the quality of its input data. If you can meticulously remove conscious and unconscious biases from your training dataset related to race, gender, and other ideological factors, you could create an AI system capable of making unbiased, data-driven decisions.
However, in reality, this is a difficult task. AI's learning is based on the data it receives, which is generated by humans. Humans, prone to biases, create the data, and the continual identification of new biases adds to the challenge. Thus, achieving complete impartiality in both humans and AI may be unattainable. Since humans produce the skewed data, and humans and human-made algorithms verify it, completely eliminating biases from AI systems is an ongoing challenge.
Nonetheless, combating AI bias is possible through rigorous testing of data and algorithms and adopting best practices in data collection, usage, and AI algorithm development.
As artificial intelligence (AI) advances, its impact on decision-making grows. AI algorithms, used in areas like medical information and policy, can significantly affect people's lives. Consequently, understanding and addressing biases in AI are crucial.
This article suggests several solutions, including testing algorithms in real-world scenarios, considering counterfactual fairness, adopting human-in-the-loop systems, and reforming science and technology education. However, these solutions may not fully resolve AI bias and could require a multidisciplinary approach. To combat bias effectively, it's essential to rigorously assess both data and algorithms and adhere to best practices in data handling and AI algorithm development.