Machine learning technology? Explained for beginner by Digimagg
Discover the world of machine learning technology, its applications, algorithms, and impact on various industries.
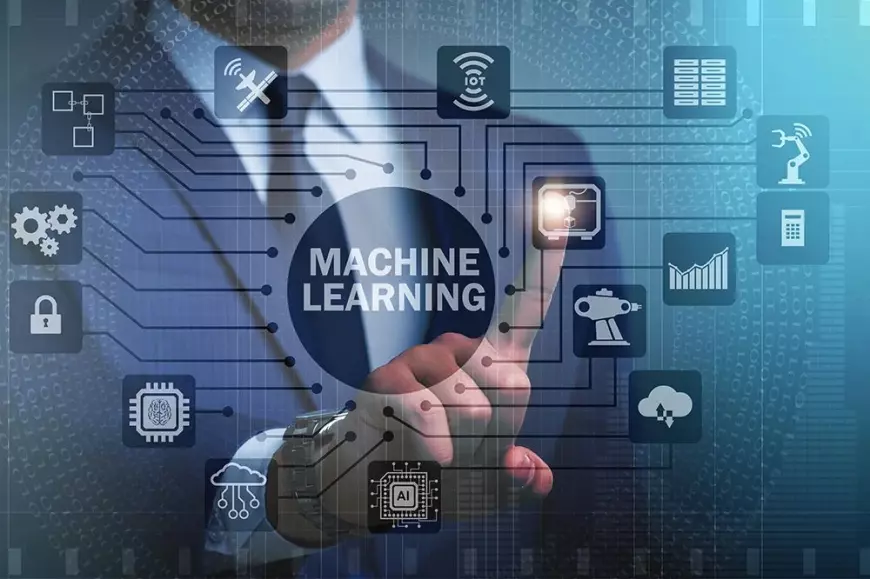
What defines machine learning?
In machine learning, systems can "learn" by analyzing data, statistics, and trial and error, enhancing efficiency and innovation. This capability empowers computers with human-like learning, addressing complex global challenges. Unlike traditional programs relying on explicit knowledge, machine learning grants computers tacit knowledge, derived from experience and context. This type of knowledge, difficult to convey through conventional means, aids tasks like facial recognition, which relies on personal recognition rather than describable rules. Machine learning's departure from extensive coding enables computers to leverage tacit knowledge, facilitating pattern discovery and prediction. Its widespread adoption spans industries, revolutionizing processes from finance and weather forecasting to governance.
How does machine learning operate?
Machine learning aggregates input data from diverse sources, including training sessions and repositories like .gov websites or Amazon Web Services. This data functions similarly to human experiences, providing historical context for machine learning models to draw upon in making future decisions.
Algorithms then scrutinize this data, identifying patterns and trends essential for making precise predictions. Consequently, machine learning extrapolates insights from past occurrences to anticipate future events. Typically, the accuracy of predictions improves with larger datasets fed into machine learning software.
The objective is for machine learning algorithms to execute tasks autonomously, minimizing human intervention. This accelerates processes across various industries as machine learning increasingly automates numerous functions.
What is deep learning and how does it work?
Deep learning, a subset of machine learning, is gaining popularity for its capacity to derive features from data. It utilizes Artificial Neural Networks (ANNs) to extract higher-level features from raw data, drawing inspiration from human biological information processing. Computer learning is termed "deep" due to its layered approach to interpreting raw data.
For instance, deep learning is invaluable in image processing across various fields like e-commerce and medical imaging. Google employs deep learning in its programs to discern patterns in images, enhancing search result accuracy. In medical domains such as radiology and pathology, deep learning's tacit knowledge, acquired from analyzing numerous scans, swiftly identifies diseases or injuries, streamlining diagnostic processes and reducing costs for healthcare facilities.
Varieties of machine learning
Just like other AI systems, machine learning requires diverse methods to set parameters, actions, and outcomes. Machine learning programs come in various types, each exploring different options and assessing various factors. These types vary based on factors such as data size and diversity. Below are some of the most common types of machine learning, categorizing popular algorithms.
Semi-supervised learning simplifies data labeling using a limited sample
Semi-supervised learning lies between unsupervised and supervised learning approaches. Instead of providing a program with entirely labeled data or entirely unlabeled data, this method offers a mix, accelerating the learning process and enhancing accuracy. Typically, programmers input a small set of labeled data along with a large portion of unlabeled data, allowing the computer to utilize the structured groups to categorize the rest. Labeling supervised data is deemed labor-intensive due to high expenses and extensive time commitments.
To differentiate between machine learning types: Supervised learning resembles being under constant supervision by a teacher, while unsupervised learning involves self-discovery. Semi-supervised learning is akin to receiving a lesson followed by relevant testing. Each type has its pros and cons, tailored to the requirements and criteria of data scientists or engineers.
Accelerated examination of complicated data through unsupervised learning
Unsupervised learning operates on input data devoid of labels and structures it by clustering or grouping. It relies on unlabeled past test data to categorize raw data based on similarities or differences. This technique, such as cluster analysis, is employed in various fields like genetic research and social media community formation.
Examples and Applications of Machine Learning
Social Media
Social media companies utilize machine learning for two primary purposes: fostering a sense of community and combating malicious activities. Machine learning achieves the former by analyzing user preferences such as pages, tweets, and topics of interest to suggest similar content or community pages. This process essentially leverages individual preferences to fuel social media recommendation systems.
To address the dissemination of misinformation in politics, social media platforms employ machine learning to swiftly detect harmful patterns of false information, identify malicious bots, review reported content, and take appropriate actions such as deletion when necessary. These efforts aim to cultivate online communities grounded in truth.
Financial Services
The financial services sector is embracing machine learning for its remarkable ability to accelerate processes with a high degree of precision and efficiency. Tasks that previously required hours, days, or even weeks to complete manually can now be accomplished in minutes. In 2021 alone, card brands like American Express processed over 581 billion transactions. To enhance transaction security, American Express has adopted machine learning to detect instances of fraud and other digital threats.
Moreover, lending and credit card companies utilize machine learning to assess and forecast risk. These computational models consider an applicant's credit history, as well as numerous other factors such as cell phone bills and rent payments, to evaluate the risk profile for the lending institution. By incorporating additional data points, lenders can extend loans to a broader spectrum of individuals who may have been ineligible using traditional assessment methods.
In the realm of trading, firms leverage machine learning to analyze vast datasets and identify optimal price points for executing trades. Sophisticated high-frequency trading algorithms consider thousands, if not millions, of financial data points to facilitate the buying and selling of shares at opportune moments.
Retail and E-commerce
The retail sector relies on machine learning to enhance sales strategies and gather insights into personalized shopping preferences. By analyzing user interactions such as clicks, likes, and previous purchases, machine learning enables retailers and online platforms to offer tailored product recommendations. This personalized approach fosters customer loyalty, as shoppers feel understood by the retailer and are more inclined to make additional purchases.
Visual search is emerging as a significant aspect of the shopping journey. Instead of inputting text queries, customers can now upload images to convey exactly what they're seeking. Machine learning algorithms analyze these images through layers, generating search results based on visual cues.
Furthermore, machine learning aids in forecasting customer trends and behaviors. By examining individual purchase patterns comprehensively, these systems can identify popular products and anticipate future trends. For instance, if a new food item gains popularity as a "superfood," a grocery store's machine learning systems may detect increased purchases of that product and subsequently offer customers coupons or targeted advertisements for related items. Similarly, analyzing past purchases enables systems to send personalized coupons tailored to individual preferences.
Healthcare
The healthcare sector utilizes machine learning to streamline medical data management, advance treatment discovery, and enhance disease detection and prediction. With machine learning-powered computer systems, healthcare professionals can access patient medical records swiftly, eliminating the need for manual file searches or extensive communication within hospitals. Updated medical systems enable instant retrieval of relevant health information for each patient.
AI and machine learning technologies automate tasks such as maintaining health records, patient follow-ups, and insurance authorization, contributing to a significant portion of healthcare cost reduction.
In drug development, machine learning collaborates with researchers to expedite the generation of drug treatments. By analyzing millions of data points, these tools identify successful compound configurations, accelerating drug discovery timelines from months to weeks.
Machine learning significantly enhances disease detection and prediction accuracy. Radiology and pathology departments worldwide employ machine learning algorithms to analyze CT and X-ray scans for disease identification. Some advanced systems, trained on vast datasets using supervised, unsupervised, or semi-supervised models, surpass human capabilities in disease diagnosis rates. Additionally, machine learning aids in predicting outbreaks of deadly viruses like Ebola and Malaria, with the CDC leveraging these technologies to monitor flu virus instances annually.