How AI contributes to carbon emissions and challenges Net Zero goals
Learn how AI's growth increases carbon emissions, posing challenges to achieving net zero targets in environmental sustainability efforts.
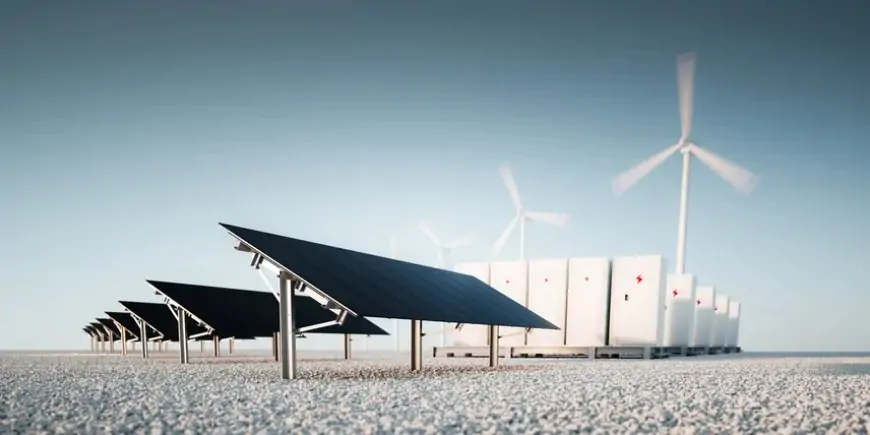
AI, known for its high carbon footprint, poses a threat to Net Zero emissions goals as companies invest in carbon-intensive models and infrastructure. A recent Google report disclosed a 48% rise in greenhouse gas emissions over five years, attributed to the energy demands of AI. The increase in data center energy consumption and supply chain emissions were major factors. The report cautioned that integrating AI into products could hinder emission reduction efforts due to higher energy demands and increased emissions from infrastructure investments. Google acknowledged that its focus on innovative AI-driven products like Gemini and Veo could sideline its green initiatives.
The energy intensity of AI: Understanding its carbon footprint
AI is inherently energy-intensive, particularly during the training and development of machine learning algorithms and models. Simple tasks like generating an image can consume as much energy as charging a phone. As models grow larger with more parameters, their energy demands escalate. Training these models involves processing vast datasets, often requiring deployment on servers equipped with multiple GPUs in data centers. These data centers, consuming 1-1.5% of global electricity, also necessitate substantial energy for cooling systems. Thus, the carbon footprint of AI stems from both server power consumption and the energy used for cooling.
Variability in energy sources: Assessing AI's environmental impact
The environmental impact of AI and data centers varies significantly based on their energy sources. Data centers powered by nuclear, hydro, solar, or wind energy produce less environmental impact compared to those relying on fossil fuels.
Measuring the true carbon footprint of AI is challenging due to the lack of a standardized method for assessing emissions from AI workloads. Compounding this issue, major AI vendors like Google and OpenAI often employ opaque development practices, providing limited transparency into model training and development.
These complexities make it difficult to comprehensively gauge AI's overall environmental impact and carbon footprint. However, insights from Google's report suggest that increased investment in AI and data center infrastructure is likely to amplify carbon emissions across organizations involved in AI development.
Strategies to mitigate AI's environmental impact
Although AI contributes significantly to carbon emissions, there are effective ways to reduce its environmental impact. Beyond developing more efficient algorithms, one approach is to reconsider the use of energy-intensive air-cooling systems.
Craig emphasized the importance of addressing both computational energy needs and cooling processes in reducing AI's carbon footprint. Traditional air-cooling systems, standard in data centers, are increasingly inefficient as computational demands grow. They struggle to dissipate heat from densely packed servers, resulting in higher energy consumption and environmental impact.
Dorian Shimy, CEO of FutureFund, suggests that processing AI across multiple decentralized data centers can lower overall energy consumption. This approach distributes computational tasks across smaller, more efficient data centers closer to data sources. It not only reduces energy lost in data transmission but also enables the use of local renewable energy sources impractical on a larger scale.
These strategies mark a departure from conventional data center operations, offering significant potential for reducing AI's carbon footprint.