Researchers utilize AI to discover new Alzheimer's risk factors
The research underscores the efficacy of machine learning in aiding scientists to gain deeper insights into the intricate factors behind diseases Alzheimer.
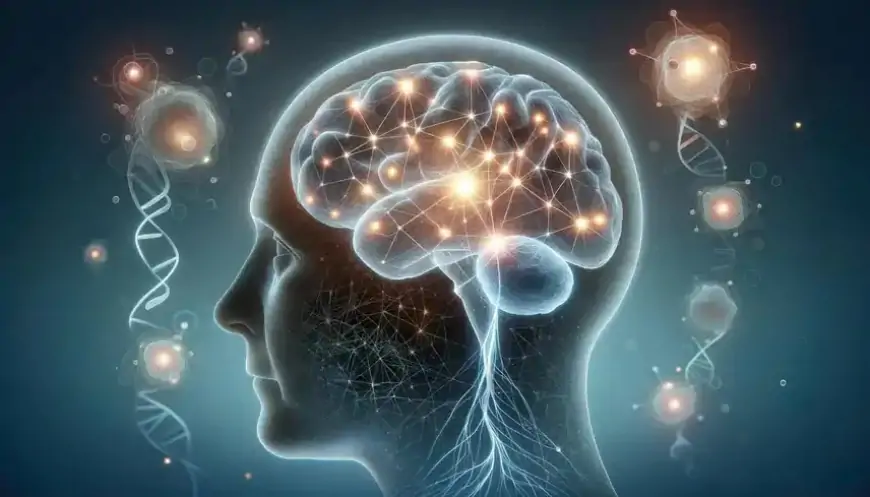
Neuroscientists possess a solid understanding of key Alzheimer's risk factors, encompassing genetics, physical activity, educational attainment, and social engagement levels.
However, a potential benefit of AI in healthcare lies in its ability to identify less apparent connections that may elude human observation. Can AI aid in discovering previously overlooked conditions associated with Alzheimer's?To investigate, Marina Sirota and her team at the University of California San Francisco (UCSF) utilized a machine-learning program on an anonymized electronic health records database. The AI was trained to identify common features among individuals diagnosed with Alzheimer's over a seven-year span. The database contains clinical information, including lab results, imaging tests, and medical condition diagnoses. Sirota notes that while some findings aligned with existing Alzheimer's knowledge, others were new and intriguing. The outcomes were documented in Nature Aging.
Conditions like heart disease, high cholesterol, and inflammatory issues have been identified as Alzheimer's risk factors, which aligns with their known role in the formation of brain protein plaques. Unexpectedly, osteoporosis in women and depression in both genders were also observed. The researchers, led by Alice Tang, a medical student in bioengineering, and Marina Sirota, emphasize that these factors don't guarantee Alzheimer's development but could serve as indicators for patients to address and potentially reduce their risk. Tang notes that detecting such factors provides hints of an impending Alzheimer's diagnosis, with modifiable aspects like high cholesterol and osteoporosis amenable to treatment.
The study was not specifically structured to ascertain whether addressing these issues could effectively reduce the risk of developing Alzheimer's. Marina Sirota and her team intend to further analyze the health records database to investigate whether individuals undergoing treatments for conditions like osteoporosis or high cholesterol eventually exhibit a lower Alzheimer's risk compared to those with the conditions but no treatment. Sirota states, "Retrospectively examining treatment data in electronic medical records is a potential avenue to explore if existing therapies can mitigate risk."Tang conducted an investigation into genetic elements linked to conditions such as high cholesterol, osteoporosis, and Alzheimer's to deepen the understanding of their interconnections. The correlation between cholesterol and Alzheimer's is influenced by the ApoE gene, specifically the ApoE4 variant known to elevate Alzheimer's risk. Tang also pinpointed a gene associated with both osteoporosis and Alzheimer's, suggesting a potential new focus for research and treatment development.
The research underscores the efficacy of machine learning in aiding scientists to gain deeper insights into the intricate factors behind diseases like Alzheimer's. Additionally, it highlights the capability of machine learning to propose innovative avenues for potential treatments.